GTC 2024 Announcements
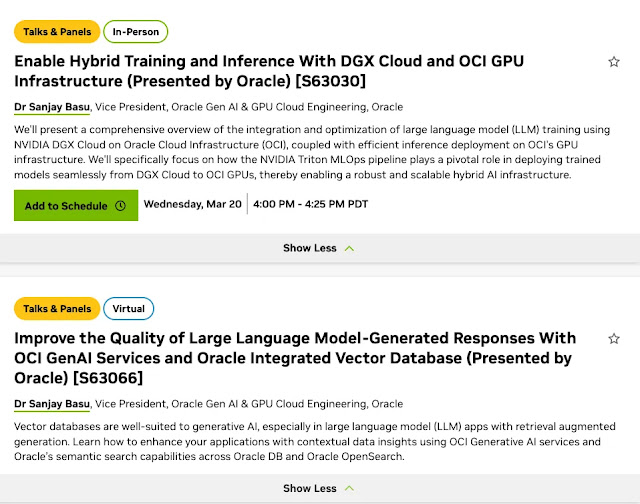
I had two sessions At the GTC 2024 event, a significant announcement was the unveiling of the new Blackwell GPU architecture, designed to usher in a new era of AI and high-performance computing. This architecture introduces several key innovations aimed at dramatically improving AI model training and inference speeds and energy efficiency. One of the highlights of Blackwell is its design, which comprises two “reticle-sized dies” connected via NVIDIA’s proprietary high-bandwidth interface, NV-HBI. This connection operates at up to 10 TB/second, ensuring full performance with no compromises. NVIDIA claims this architecture can train AI models four times faster than its predecessor, Hopper, and perform AI inference thirty times faster. Moreover, it reportedly improves energy efficiency by 2500%, albeit with a significant increase in power consumption, up to 1,200 watts per chip. The Blackwell architecture brings forward six primary innovations: an extraordinarily high transistor count,